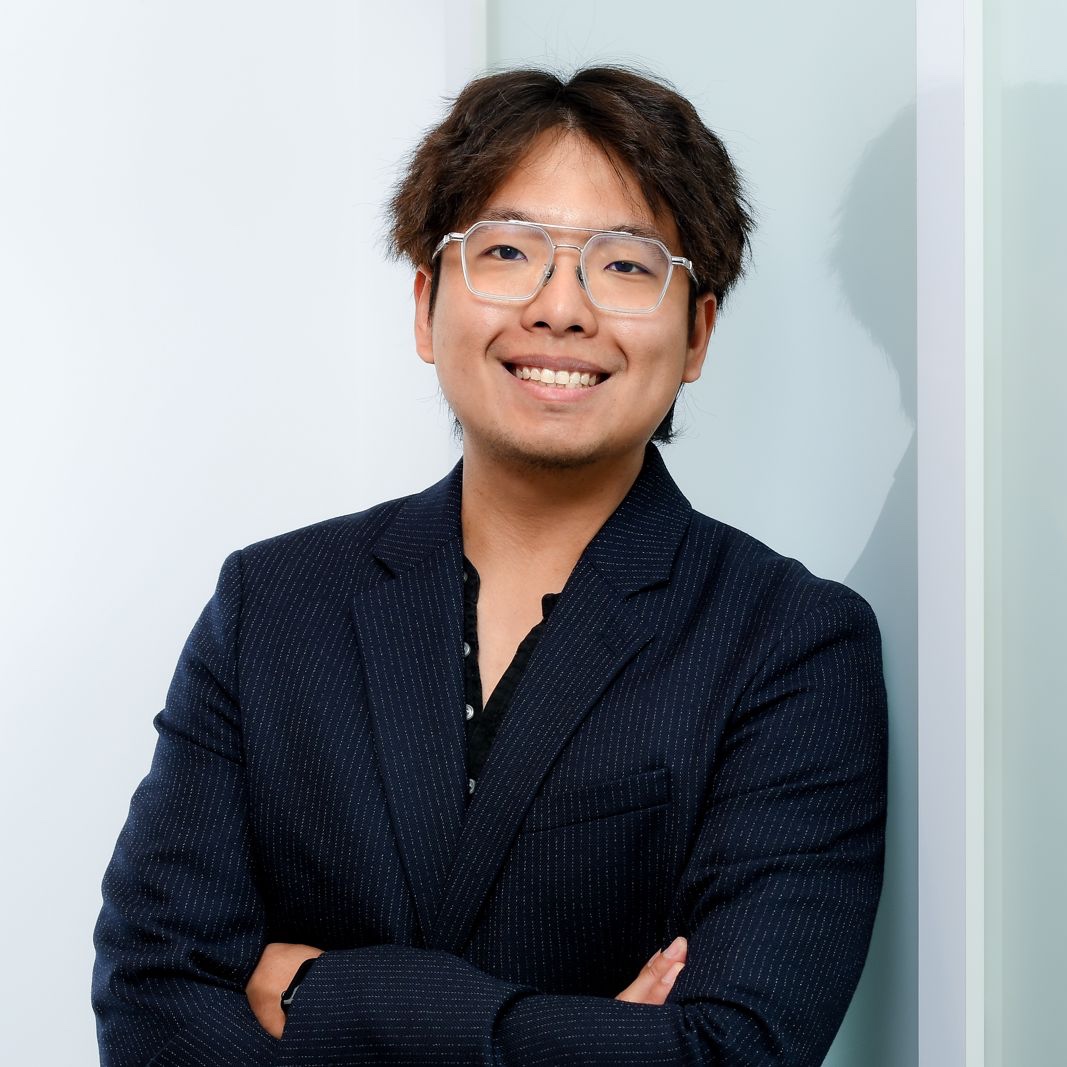
🚨🚨 PhD Opportunities: Interested in bringing in epistemic intelligence to machine learning systems? I have several openings for PhD students to start in Jan 2026 to be based in Singapore and are looking for candidates with a strong mathematical, statistical, or machine learning background. More details can be found here.
Welcome
Hi, my name is Siu Lun (Alan) Chau. I am an Assistant Professor in Statistical Machine Learning at the College of Computing and Data Science, Nanyang Technological University (NTU), Singapore. I lead the Epistemic Intelligence & Computation (EPIC) Lab, where the mission is to develop intelligent systems that can recognise their own limitations and communicate what they know--and how well they know it--with clarity and confidence. To achieve this, we focus on the theoretical foundations and methodological development of epistemic uncertainty-aware machine learning. In particular, we leverage tools from imprecise probabilities, cooperative game theory, and kernel methods to represent, quantify, and resolve uncertainty, as well as to support learning and decision-making under uncertainty.
Background. From 2023 to 2025, I was a Postdoctoral Researcher at the Rational Intelligence Lab within CISPA Helmholtz Center for Information Security, Germany, under the supervision of Dr. Krikamol Muandet. My research centered on imprecise probabilistic machine learning, a generalised probability theory framework, and their integration to machine learning challenges, including hypothesis testing, supervised learning, and forecast elicitation under imprecision. Between 2019 and 2023, I pursued my DPhil in Statistics at the University of Oxford, where I worked at the intersection of kernel methods and Gaussian processes, under the supervision of Prof. Dino Sejdinovic. During my doctoral studies, I also interned at the Empirical Inference department of the Max Planck Institute for Intelligent Systems, Tübingen, working on improving econometric models using modern machine learning techniques. I hold both a Master’s and a Bachelor’s degree in Mathematics and Statistics, graduating with First Class Honours from the University of Oxford, where I received the Departmental Prize for ranking top of the cohort.
I also enjoy bridging the gap between academia and industry. I interned at Amazon as an Applied Scientist, addressing coherent forecasting problems for the EU logistics network. I also provided data science and machine learning consulting services for various startups, including Ravio, Catalyst AI, Cambridge Spark, Crop4Sight, and gini. I also co-founded Oxford Strategy Group Digital, Oxford’s first student-led data science consultancy group.
News
- May-2025
I will be presenting Credal Two-sample Testing at ISIPTA 2025! Excited to share this work with the Imprecise Probability community!
- May-2025
Our paper on Truthful Elicitation of Imprecise Forecasts has been accepted at UAI 2025 as an Oral paper!
- May-2025
Our paper “Kernel Quantile Embeddings and their Associated Probability Metrics” has been accepted at ICML 2025!
- Jan-2025
Our paper on the first ever Credal Two-sample Testing has been accepted at AISTATS 2025! Many thanks to the amazing crowd of collaborators.
- Nov-2024
I will be starting my Assitant Professorship at the College of Computing and Data Science at Nanyang Technological University in Singapore in May 2025.
- May-2024
Our paper Domain Generalisation via Imprecise Learning has been accepted as a spotlight paper for publication at the International Conference on Machine Learning (ICML) 2024! 🎉
- Feb-2024
I presented “Stochastic Shapley values for Gaussian process models with application to Explainable Bayesian Optimisation” at the Institute for Informatics, LMU.
- Feb-2024
I was invited to participate in the Dagstuhl Seminar on ‘AI for Social Good’ this year to learn about and tackle challenges faced by NGOs when implementing AI solutions.